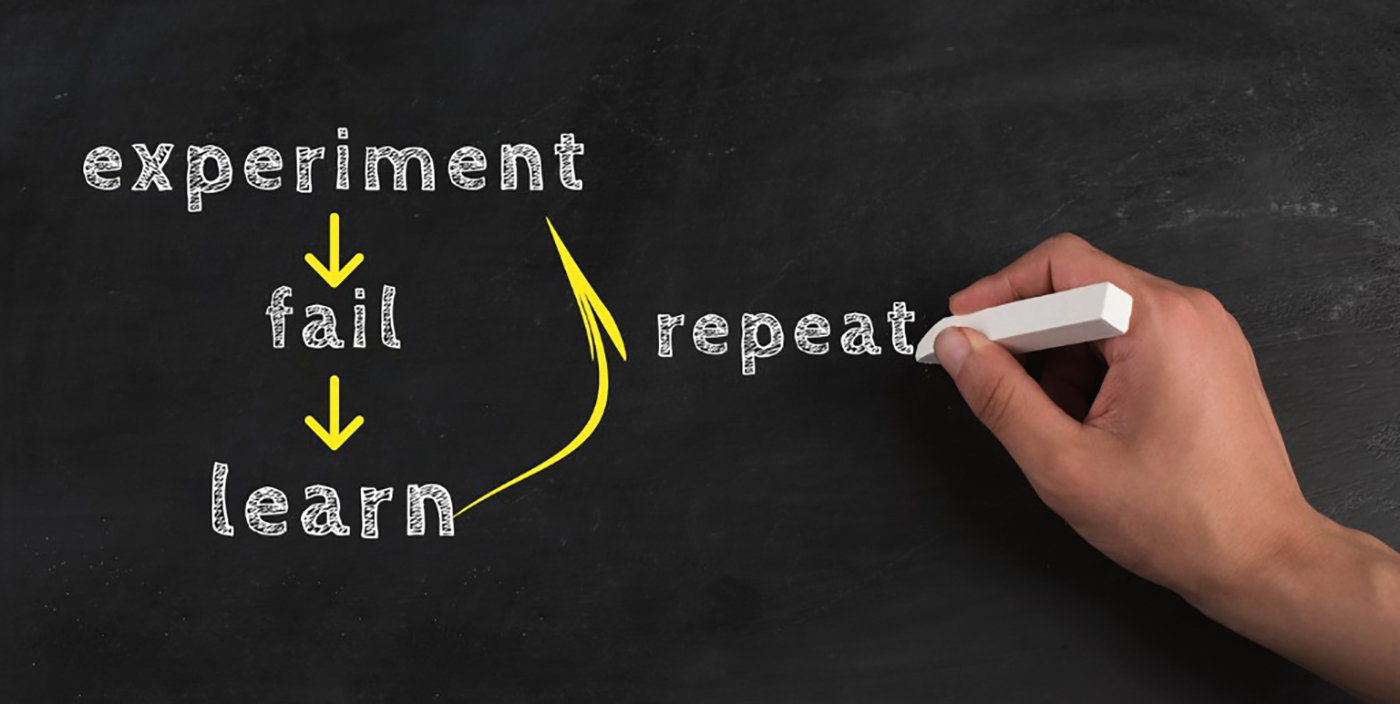
It’s been a little over a year now since I shared in my previous article how we were approaching the design of a Monitoring and Recovery System to help one of our key clients diminish the impact and severity of unplanned disruptions and minimize the downtime of one of their major industrial machines.
Like most journeys, this one came with its ups and downs, from moments of high fives and milestone celebration parties to what seemed to be endless days of despair and questioning the viability of the project and if we could successfully reach the next milestone! Introducing a new system, solution or tool is a major change to the standard ways of working in any organization and we had to go through tough times where we didn’t believe we would get out of the valley of despair of the classic change curve (was more like the valley of tears in our case).
Looking at the bright side, we’ve finally reached a stage where we are starting to realize planned tangible and intangible “soft” benefits like:
- Increased uptime of the machinery that is reflected in increased productivity and profitability.
- Improved safety and reduction of accidents and injuries through the identification of potential safety issues and automatically recovering before an incident occurs.
- Reduction in maintenance costs through the identification and prevention of small problems from turning into larger, more costly issues.
- Increased staff satisfaction and engagement rates as a result of the reduction in the number of repetitive, time-consuming and non-value adding tasks that have been successfully automated.
Most importantly, what have we learned and what precautions I would take moving forward?
Being a strong adopter of agile ways of working and a firm believer in the importance of retrospectively evaluating an experience, I sat down at the start of the new year to reflect on what went well and what could we have done better as we prepare to move forward with the planning of the next product phases and identification of new opportunities to leverage digital technologies to continue delivering value to our clients.
In hindsight, I would give more focus to 3 points (maybe precautions) as we move forward:
- Invest more in the
Investing early in training and education of human capital will always pay dividends human element: I would invest more time and budget on training and education of staff, machine operators and end users to help increase buy-in and adoption of the new solution. This is especially important since the system represents a significant change to the way things have been done in the past. I would also establish clear communication channels and protocols for addressing disruptions and failures, and keep thorough documentation of the system’s configuration and performance. This can help to ensure that any issues can be quickly and effectively addressed.
- Regularly run testing and simulation: I would schedule regular, frequent testing and simulation of the system to help ensure that it is functioning correctly and can effectively handle disruptions. This could include running drills or simulations to test the system’s response to different scenarios and implementing concepts like chaos engineering (I am big fan of Netflix’s Chaos Monkey) to build confidence in the system’s capability to withstand turbulent conditions in production especially with the presence of thousands of sensors across the different subsystems where literally anything could go wrong anytime.
- Implement redundancy and failover: I would make sure we implement redundant systems that could help ensure that the automated recovery system has backup support in the event of a failure. This could include redundant sensors and control systems or other critical components. I would also make sure we lay out and implement a plan for recovering from natural disasters or power outages. This could include creating regular backups of the system’s data and configuring redundant systems to provide backup support in the event of a disruption.
Next step: AI, anyone?
While thinking of our next product releases, I couldn’t resist the temptation of squeezing AI into our plans for the coming phases. The business case for AI in the manufacturing industry has long been validated and the remarkable AI use cases in manufacturing are countless since it has the potential to significantly improve the efficiency and competitiveness of the manufacturing industry by automating processes, improving quality and optimizing the supply chain.
The business case for AI in the manufacturing industry has long been validated and the remarkable AI use cases in manufacturing are countless …
Here are 3 ways/areas at a high level where I believe AI could be leveraged in the implementation of the next major release of our monitoring and recovery system:
- Predictive maintenance: where
AI-powered predictive maintenance helps keep machines running smoothly by predicting and preventing potential issues before they occur. we can use machine learning algorithms to analyze data from sensors in order to predict when equipment is likely to fail or need maintenance. This data can include factors such as vibration levels, temperature, and lubrication levels. This can help us further reduce downtime and improve the overall reliability of the machinery.
- Fault diagnosis and classification: where AI helps with the analysis of data from sensors and other sources to identify patterns and trends that may indicate a fault or malfunction. It can then classify the fault based on its severity and impact, and accordingly prioritize repairs and minimize the impact of disruptions on the overall operation. On the flipside, AI algorithms can be used to analyze the same data to identify patterns and trends that may indicate opportunities for optimization or performance improvement. For example, an AI system might identify that a particular piece of equipment is running at an inefficient speed, and recommend adjusting the speed in order to improve efficiency.
- Natural language processing: Natural language processing (NLP) is a subfield of AI that focuses on enabling computers to understand and interpret human language. We can think of leveraging NLP to interpret and respond to maintenance requests helping to streamline communication and improve the efficiency of the recovery process. For example, NLP could enable human operators to interact with the automated monitoring and recovery system using natural language which would make the system more user-friendly and improve the speed and accuracy of communication between operators and the system.
The takeaways in brief: implementing an automated monitoring and recovery system can be a valuable investment for industrial machinery, but it’s important to carefully consider the precautions discussed above and learn from our experiences in order to maximize the benefits and minimize potential risks that come with the introduction of major change initiatives. On other critical note, AI is rapidly becoming the go-to technology in almost every industry and its use cases have become endless. Business leaders should seriously consider leveraging AI in the manufacturing industry to develop more predictive models and further optimize the performance of industrial machinery.